Data cleaning – how you can really save money
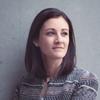
Say, for example, a member of staff has changed her position within the company. Unfortunately, there has been a failure to enter her change in cost center into the system, meaning she is still allocated to the old cost center – it’s an accounting error.
It happens. Incorrect data sets always lead to erroneous data, also called “dirty data”. In the worst-case scenario, bad data sets can block processes and shut the system down. This frustrates users and is detrimental to the organization. In some companies, data issues are generated almost methodically, as errors are entrenched in set routines and business rules. Staff are not even aware of what is happening in the process. This is especially true of companies that don't have a clear data strategy for managing information. In such cases, there is a lack of data engineering expertise and no clear, standardized data infrastructure. There’s also no effective data governance – that is to say, no holistic data management system that uses guidelines to safeguard data quality, protection and security. Yet data governance is a must these days.
Without such guidelines, staff don’t know which information they should put in which dataset of which database, never mind how they should maintain the data. Even worse, staff are often completely unaware of what information even exists in the datasets. As a result, they create a new customer address instead of conducting a cross-check to see whether it is already in the system. It’s as easy as that to create a duplicate. And that can soon become hundreds of duplicates.
Data silos are another unwanted phenomenon. These are created when individual specialist departments within a company gather and hoard information in their own datasets and databases independently of each other, for example. As a consequence, databases in one business unit swell with datasets other units know nothing about. This could be because there is not enough coordination between departments about which datasets they need in which form – or even no coordination at all.
Without data cleaning, the damage is huge
The uncontrolled growth of data – with duplicates, data silos, data that is incorrect or filed without being allocated to the right dataset – has many effects on operations. All of them negative. Organizations must reckon with the following consequences.
- Frustration among staff:
Employees spend around one third of their working time searching for data. This estimate comes from a report by consultants at McKinsey. - Incorrect analytics and results:
Errors can even be found in the master data of ERP systems. In the example mentioned above, a specific failure causes an accounting error. However, even typos can lead to additional costs, for instance if someone accidentally enters the eight hours of training they have undertaken into the learning management system twice. - Breaches of compliance regulations and data protection:
Another reason why data quality and transparency are so important is that companies are constantly having to meet new legal and regulatory requirements such as the GDPR if they are to avoid heavy fines and compensation claims. - Image damage and loss of trust:
Poor quality data can have a massive impact on a company’s reputation, including a loss of trust – for example, a badly maintained dataset could result in customers receiving the wrong product due to information being filed wrongly.
The bottom line is, data quality issues cost a lot of money – according to MIT’s Sloan Management Review, around one fifth of sales, to be precise – the IBM Group from the U.S. puts a similar figure on the overall costs. For the U.S. economy alone, this amounts to losses of around 3 trillion U.S. dollars a year.
These eye-watering sums make another figure published in the Gartner Data Quality Market Survey 2017 all the more astonishing. According to this survey, six out of ten companies have no idea how much money they are losing every year as a result of their poor data quality. Why is this? It is because they have no idea what the effects of bad data or poor data management are, so they don’t see the point in having a process to analyze and assess the consequences.
Data cleaning – five key arguments
Such an approach is fatal. Only by realizing the scale of the losses will companies be prepared to change something, for example by conducting a data cleaning process.
Data cleansing is an umbrella term that covers a range of methods and procedures aimed at deleting duplicates and correcting data that doesn’t meet certain data quality criteria. It is therefore high time to take the first step and define data cleaning as a goal, because there are many rewards for doing so.
- Data cleansing makes your decision-making more reliable.
By cleaning your data, you can ensure you have an accurate picture of reality and can make well-founded decisions on that basis. The cleaner your data, the more up-to-date the information is, and therefore the more reliable your decisions are. - Data cleansing helps boost your efficiency.
Cleaning your datasets and maintaining them to ensure they contain accurate data speeds up your business processes. For example, your sales team gains a huge amount of time if it can rely on the accuracy of customer data and entries in the CRM system and has confidence these are up-to-date and free of duplicates. - Data cleansing helps optimize your risk management.
Conducting a data cleaning process makes it possible to correctly assess all financial risks at every possible level – be it in raw material procurement or in dispatch. - Data cleansing makes your customer reach more focused.
The more up-to-date your datasets are, the more accurate your customer reach will be. With a dataset that uses clean data, the stage is set for you to offer your customers the right product at the right time. - Data cleansing ensures compliance.
Data cleaning lays the foundation for data integrity. You ensure that your data is always correct, free of duplicates and can be used reliably. This is the only way you can meet your documentation obligations in full.
Data cleaning – best start now, and do it methodically
However, when is the best time for data cleaning? Of course, the best thing is to never need to embark on data cleaning in the first place because you have kept clean data from the outset. If you haven’t done that, though, you should immediately go about putting things right. The earlier a company undertakes data cleaning, the better. Unstructured data is expensive and risky from a data protection perspective – not least when it comes to moving to the cloud.
Data cleaning should therefore be a matter of concern at the highest level of every company. This includes equipping the people responsible with the necessary skills and ensuring close coordination between everyone involved when it comes to maintaining data. After all, once a data analysis has been performed, the bad data highlighted in the analytics has to be identified and corrected, data volumes reduced and potential data sources consolidated. In certain circumstances, it may be necessary to transfer the data to new databases – offering a great opportunity to standardize processes at the same time.
In this age of digitalization, of course, time-consuming manual data cleaning is very much a thing of the past. Cutting-edge solutions and software tools for data cleaning perform a valuable service. They operate based on context, so just a small amount of key data is enough to automatically consolidate and integrate the data collected in the company.
For the example we used at the beginning, that would mean the company would only have to provide three key data points to clean the data – the name, the old cost center and the new cost center – rather than a comprehensive template with 30 to 60 data fields. The tool takes care of the rest in an automated process. Sometimes, cleaning up isn’t so bad after all.