LERNLUST #53 // If AI knew what the expert knows...
Show me similar content
LERNLUST Podcast Knowledge Transfer Knowledge Management Artificial intelligence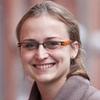
In today's episode, we address an issue that is central to many organizations: securing and making knowledge available. A shortage of skilled workers and increasing digitalization are challenging organizations to capture the valuable knowledge of their employees and make it available for the future. Traditional methods such as personal interviews or workshops are often too costly and do not deliver the desired value.
In addition, the results in the form of stored text documents often represent inert knowledge and are therefore not very effective in terms of application.
AI offers us new ways to automate the questioning and externalization process and make it economical. This applies both to the questioning itself and to the subsequent sequencing and processing of the collected knowledge.
How are we realizing this vision together with our colleagues from Frauenhofer FOKUS and what is behind the technical innovations? We discuss all this and much more in this podcast episode.
Shownotes
Host:
Susanne Dube, Teamlead Learning // LinkedIn
Gast:
Max Upravitelev, Research Associate, Frauenhofer FOKUS // LinkedIn
Katharina Vögl-Duschek, Senior Learning Consultant // LinkedIn
Learn more about the tts knowledge safe
https://one.tt-s.com/en/tts-knowledge-safe
You can also find all episodes of our LERNLUST podcast at:
Apple Podcasts | Spotify | Google Podcasts | Amazon Music | Deezer
Transcript
Intro
LERNLUST, the podcast for everything related to corporate learning. We are Susanne Dube and Claudia Schütze and we are learning consultants at tts. It's great to have you with us today.
Katharina
What we also experience very, very often is that due to this current lack that prevents us from conducting an expert debriefing, even when expert debriefings do take place, there is often the problem that the right questions are not asked.
Susanne
What happens to the knowledge after the conversation? Now you have a system, ideally at some point you will have managed to ask the questions in such a way that it can access the experiential knowledge. And what does it do with the experiential knowledge?
Max
And these are all nice technical problems that you can then tackle with it.
Susanne
As a learning consultant, I usually deal with helping people learn, with training concepts, learning materials, learning support and all my experience. But a very different question has been on my mind and that of many enterprises for a long time when it comes to skills and knowledge. How can I retain knowledge and skills in the enterprise? The advent of artificial intelligence has given some of my colleagues completely new ideas here. Today I am talking to Max Upravitelev and Katharina Vögll-Duschek about what these are and how we complement each other with the partners of the Fraunhofer Institute. Katharina has often been our guest here on the podcast. On today's topic, she has already raised the question of possibilities for knowledge externalization during her studies. She is currently focusing on the distinction between declarative and procedural knowledge. This is because it brings us a step closer to the actual knowledge of how to act. And that is what enterprises want to hold on to. But research is not the core of tts. That is curiosity. So it's no wonder that our team has sought support. Max is currently working at Fraunhofer Fokus. His focus there is on the application of LLMs, or Large Language Models, within learning technologies. He actually comes from the humanities and worked in the cultural sector. But then he was gripped by a desire for computer science. And for his specialty, machine learning. Both Max and Katharina are here today to tell me about their AI project, the Knowledge Safe. And I can't shake the feeling that I'm learning a lot today. Welcome, dear Max. Welcome, dear Katharina, to the LERNLUST sofa. I'm really happy to see you, because you've brought me such a fascinating topic. Are you both doing well today?
Katharina
Yes, I'm fine. I hope you are too, Max.
Max
I'm doing very well.
Susanne
Absolutely excellent. I think that's really excellent. It's also a Friday afternoon, I have to say. We've all had a week. Nevertheless, as I said, we've chosen a nice topic. It's called Knowledge Safe. Now Knowledge Safe can mean a bit of everything and I'll give you a little spoiler. It has something to do with AI and that's exactly why I, Susanne, became curious about it. But before I say something wrong and describe it in a way that goes completely against the grain for you, I'd like to know what the Knowledge Safe is. What does it actually mean and how did you come up with the idea of building a safe for knowledge?
Katharina
Yes, then maybe I'll start with that. And Susanne, exactly, you've actually already addressed that quite nicely, a safe for knowledge. Actually, it's a very good term, because “Knowledge Safe” means exactly that. And that was actually the basic idea at the time, which came about during an internal tts strategy workshop on artificial intelligence where we had simply started to look at AI topics and the potential that AI offers for solving classic customer problems. And that's actually how we came up with the idea in the first place. And that means nothing more than that we want to use it to really store knowledge from enterprises and organizations in the long term. And then to make it available again in the long term. That means safe in the sense of storing it clearly, but actually also making it available again.
Susanne
Okay, so does that have something to do with knowledge management or what do you mean by that? How do you make it available? Simply from any old source?
Katharina
Exactly, so knowledge management, yes, that's actually a good description. The basic idea is really to make knowledge from experienced employees, so to speak, in particular this experiential knowledge, tangible, explicit and secure for the general public. So, in very specific terms, we came up with the idea that, in the case of off-boarding, when an employee of many years leaves the enterprise, there is of course a lot of knowledge in the employee himself and we all know, I think, from different experience that off-boarding often doesn't go as smoothly as one would like, and that's where the Knowledge Safe comes into play explicitly. But that doesn't mean that it should only be focused on the off-boarding process.
Susanne
That means you are actually hinting at the issue of the shortage of skilled workers, in that you say that the skilled workers who may leave the enterprise should at least leave their knowledge there. That's a bit of it, if I understood that correctly. But not only that, but whenever someone is very knowledgeable, we want to pick that knowledge out. I'm very, very curious to know what that means in detail. But let's be honest about knowledge management. When I was at university, it somehow already existed in 2001. There were already competence centers that dealt with it. So this question of how to keep knowledge in an enterprise has been around for a long time. So others have had an idea similar to yours of building a knowledge safe?
Katharina
Yes, definitely, there have been some ideas along those lines, especially with the advent of AI. I think that later on, when we've got it all a bit more sorted out, we can go into more detail about what we've observed, including the similarities and differences. But of course there are a number of issues that were simply difficult to solve in the past, which is why new possibilities are now emerging through AI, such as lack of time and so on.
Susanne
Okay. So that means there has been another boost and now everyone is getting active. And then suddenly it happens that tts is working together with the Fraunhofer Institute. Max, maybe that's a question for you too. How is it actually that the Fraunhofer Institute is working with tts on this topic?
Max
Well, to be quite specific, I work at the Frauenhofer Fokus Institute here in Berlin and we have a department here that deals with various media applications. And within this department there is a working group of which I am a member, which in turn deals with learning technologies. And that's how the actual contact with tts came about. And there my specific area is again everything that concerns various AI, so I'll call it for the sake of freedom, AI models and algorithms. And above all, I'm currently working a lot with language models, in other words with the large language models. That means it was just a perfect fit and for me personally it just sounded like an extremely exciting research project. So, to find out how to actually implement what was demonstrated.
Susanne
So, now let's get down to brass tacks. We've heard a bit, okay, it's about capturing people's knowledge. It's maybe a bit about knowledge management, a bit about the shortage of skilled workers. You're now talking about Large Language Models and various terms that people outside the industry might not even understand yet, because what you do sounds very technical. So it also has something to do with AI. Now explain exactly what the Knowledge Safe does. How is it implemented? What should I imagine? Describe it in a little more detail. Yes, I'd like to get started. So maybe I'll start with the point that was somehow my logical anchor for all of us, namely this, what problems are we actually solving or what should this aim for? Well, we already talked a bit about it at the beginning, that this classic lack of time is often a problem with offboarding. So that's just the one anchor point where we tie in, that we just say that we want to make offboarding structured by ultimately conducting structured interviews with experts and simply conducting the expert debriefing in a more structured way.
Susanne
Okay, a guideline is enough for now, but that wasn't enough for you. Exactly.
Katharina
What we also experience very, very often is that due to a lack of time, which prevents us from conducting an expert debriefing, even when it comes to expert debriefings... often the problem is that the right questions are not asked, so to speak, that one is not specifically looking for procedural knowledge, but often remains very superficial and just wants to ask about the loose knowledge building blocks, which are then not necessarily aligned with the position that the person had and certainly does not get to the deeper procedural knowledge or experiential knowledge. And even if you managed to do that to a lesser or greater extent, the next hurdle was always to make this knowledge available so that the rest of the organization could somehow work with it and do something with it. And of course a lot of that knowledge was perhaps lying around in finished interview guides or in transcriptions and was then simply, it was just classic inert knowledge that you couldn't really use. And from all these components, the very, very simple idea arose, I would say, if you look at it purely from the meta-level, to say, we want to use structured interviews to interview experts multiple times, so to speak, and to ask about the knowledge in a targeted way. And this interview process should be completely AI-controlled, so to speak, and controlled by the AI in such a way that it always finds the right follow-up and follow-up questions and can ask them.
Susanne
And I find that really exciting. So just to summarize: you want to get directly to the experiential knowledge of a person with clever interview questions that an AI asks in such a way that they are always adapted and not just a simple guide, and you want to make the answers available to the rest of the world.
Katharina
Right. That's the whole basic principle.
Max
So I find exactly this point, also technically, so incredibly exciting about this project, because it hits the nail on the head, which is also so difficult to implement. Because the language models, or rather what is generally understood in AI, are actually trained to give answers, not questions. And that's exactly the opposite. So it's about asking questions. And not just any questions, but good questions. And good also in the sense of several possible metrics. So these have to be questions that feel good. That is, where a person already has the feeling that someone is talking to them. So that means there has to be some kind of feedback with what has been said so far, so that it's not, so to speak, a rattling off of predetermined questions, but that it's really a conversation. And that just has to do with the job. So, quite banal, broken down. So it takes a lot of contextual knowledge that has to be included. And these are all nice technical problems that you can then tackle with it.
Susanne
That sounds so nice when you say it. These are nice technical problems. Actually, maybe you could elaborate on these sticking points again. So that's a pretty broad thing you mentioned. So in the beginning you need the basics. You said that contextual knowledge has to be there somehow, the system has to know that. How do you do that? So do you already have an idea?
Max
Well, what we want to tackle above all is the challenge that large language models can do an extremely wide range of things. They can generate things, which is extremely cool, and the things they can do would have been unthinkable before, but they can't plan. And something like an interview plan is anything but a trivial task, at least from a computer science perspective, because there are just so many possibilities when it comes to formulating a really good question. And one approach we are pursuing, for example, is to work with documents, especially with regard to this contextual knowledge. And that is possible in a situation, for example... well, I usually imagine a bit different situations, I usually just imagine something like, we have just completed a project and are currently evaluating it. So, that, so to speak, can also be a tool that doesn't react to staff turnover, but simply to something like evaluating the results. And a lot is produced there, as a rule. So, there are reports, there may be important e-mails and stuff like that, there are minutes from evaluation discussions, whatever. And all of that can be fed into a system like that using a wide variety of techniques. And then set that again as a context, which means that I then have a very, very specific context from a conversation. So before the actual conversation has even started.
Susanne
Exactly, so then I have the context. But now you have also said that you then want to use the knowledge and experience of the people. So you are not only interested in the documents, but also in what a person might do with them. So maybe an example, when I create training courses and things like that, I have processes, I might have needs assessments and all sorts of things, but you want to get at what I have inside me, the way I maybe approach the needs analysis, how I might ask what notes I take from it, how I process the answers, maybe generally weights that I do in my job, you want to get at this knowledge. How do you do that then? That's the tricky part, asking questions, right?
Max
Absolutely. So that's the part that's really the most fun, because that's exactly what it's about. So that's basically the biggest challenge. Even we already have some contextual knowledge that people have created themselves. We use that as a starting point to ultimately formulate questions that make them think about things they might not have thought about yet. So that's the real added value. That it's a tool that helps people come up with new ideas. And that's exactly where it gets interdisciplinary, because that's been a big topic in philosophy for thousands of years: how can I know what I don't know?
Susanne
Yes, exactly.
Max
So that's basically the big crux of the whole thing.
Susanne
Okay, good. And can you already tell us a little bit about how you do it, how it is solved? Or is that a big secret and we are not allowed to talk about it at all and you say, yes, but we have solved that and that works and we always ask the right questions now? Or what can you tell me about it?
Max
Well, I think we have two perspectives on this. And that, in turn, is another exciting thing, namely that you really have to do this in an interdisciplinary way. So from my technical perspective, my current approach is simply to ensure that the conversation has as much variance as possible. This can now be measured, also coolly, by the fact that, well, I'll just mention it briefly, but you can create embeddings of what has been said and then you can really take measurements of the meaning and then pay attention to something like that, that the conversation is as broad as possible or maybe deepened at one point and stuff like that. And then formulate the next questions accordingly. However, that's only part of it. Perhaps much more important are the questions that are added as example questions, for example. Good introductory questions and, of course, a completely different knowledge, which does not come from computer science, then again plays a very, very important role.
Susanne
You just mentioned example questions. That means you also enter example questions and the system then orients itself to them, or how did you mean that just now?
Katharina
Yes, well, as a rule, we did the same in the first few rounds, that we really just selected questions that were relatively broad, as Max said, we are very much concerned with looking at the broad picture on the one hand, but then also at the depth, at the point where it is then appropriate for the depth, to get there. And so our approach was to start with a broad range of questions and then narrow them down. We identified a pool of questions that would help us to get through the initial exploration. Of course, always with the ulterior motive that we have the data analysis in advance and also already have a great deal of background knowledge about the people per se, through the job description and also through perhaps preliminary interviews that one then conducts with the test subjects. And so the question must not be too broad, because of course the people don't want to be interviewed a hundred times and they definitely want to be picked up at the point where they are.
Susanne
Yes, I mean, one goal was actually, you said that, I think, at the beginning, it's always very, very time-consuming to conduct such interviews and do the whole thing. And actually, one goal is to make it a bit... I'm not saying slim, because that would be wrong, because you tell me about variances and the questions should be broad. But to make it efficient, to ask the questions in the right way.
Katharina
In principle, yes, to stay in a tight corset, so to speak, where we are broad enough. But as Max said before, this topic of knowledge, what we know, that we can simply trigger at the right point and thus arrive at the experiential knowledge. And then just keep nudging the people until we've really gotten to the heart of the matter.
Susanne
Okay. Are there any particular areas where AI is particularly good at the moment and where it might have created a solution that could be used earlier? I mean, in the past I would have sat an interviewer there and he would have done what I'm wondering about right now with a lot of brainpower. I don't know at all. Do you have an estimate?
Max
Well, that's where we have to, well, that's basically the setting where we are right now.
Susanne
Ah, okay.
Max
Well, we have to find that out and evaluate it, and then develop and test new approaches on that basis. But that's basically exactly where we are right now. We already have the first results and they are very promising. But the big difference to a human being is precisely that. It just takes a human being. And with a system that we have built around a language model, it should be completely automated.
Susanne
Okay. That means that you are still at a crucial point where you are just doing research, a bit like that, to find out how it can work best. But you are already hoping that you can do it? Or are you saying, ah no, we'll be stuck with this for the next ten years and then at some point, when the next stage of AI development comes, we'll solve it at some point? Or how should I imagine it? I'm always such a heretic, I always ask such nasty questions, I'm sorry about that.
Max
Well, I would really love to in the next ten years. There is so much in it. There is really an extremely large amount of content in it, because it simply takes up so much. The topic of interview planning alone is simply huge. And there aren't really that many approaches to it yet. So many approaches in research have tended to work with rule-based systems, where, in turn, you don't have exactly that, which also regulates a question based on what has been said so far. So I'm still relatively at the beginning there. It's an extremely large field. You can get a lot out of it, but we don't have unlimited resources and a very specific use case. That's why I would say that it is definitely within reach, or rather what we already have can be used to work with and we are now actually at the stage of optimizing it and seeing what it will look like outside of the lab, so to speak, and how it will look when people are actually sitting in front of it and finding it all, and then you can start classic focus groups, for example, and just see how it is received and how it could be improved.
Susanne
I have to admit, I have seen a mockup like that. There is always this design technique and I once talked to a graphic designer about how it could be structured and I found it really exciting, how the questions come up and what I might be able to do as a user and go into it. You just said, yes, and then comes the testing. Is that something you are already planning or was that more of a look into the future from you? Just so I understand. So you're saying yes, yes, actually we're pretty good at this.
Katharina
Exactly, so far we have already carried out various smaller pilots and have tested this again and again, also in different stadiums. As Max has already described, it was a process that had to be developed, especially with regard to the question of automation and in connection with the semantic variance measurement. And right now, we are at the stage where we are often at when building a product: the more diverse the industries and the more diverse our target group, the better and the more different things we can try out. And yes, that's why we are simply in the process of coordinating and planning the next pilots with various enterprises.
Susanne
That's exciting. Does that mean you're already continuously testing and trying things out, getting feedback, and that's all? You just said a word. I'm sorry, Max, if I couldn't fit that into what you said before. Semantic variance measurement. Was that right? What is that?
Max
I can try to explain that briefly. In principle, it's the approach we've developed to measure how thematically broad a conversation is. So, from a technical point of view, we are constantly evaluating a conversation and, above all, we can see how different algorithms we develop can be used to generate questions and how they behave differently. Because ultimately, the exciting topic for us in development was how to actually program what should happen there. Because normally, in all possible systems that are built with LLM models, you need people. And that's the big, big problem with just about every possible technology that works with generative AI. In the end, you need people to look at it and simply say whether it still makes sense or not.
Susanne
Because otherwise they get lost and hallucinate and do other things.
Max
For example. Or they are just not good questions or whatever. And that's why, since we are currently looking for algorithms that could evaluate this again and make a conversation as broad as possible, we worked with so-called LLM personas during development. This means that we basically had two instances, each representing different people who came from tts, who were very specific, so to speak, had their own CVs, their own behavior, how they talked, how they were tuned in, how they argued, and so on. And exactly different professional fields, so to speak, had their own complete profile. And from this perspective, they also responded again and again. The development process consisted of us repeatedly conducting conversations with these personas and then evaluating how it went. And this evaluation, in turn, is where this semantic variance came from. So you can, it exists in the literature, I had read the term semantic coherence a few times. It is very important for something like the automated generation of entire works of text, something like a screenplay. Because it can easily happen that a model somehow strays, somehow in terms of content, and then that's exactly what stops working properly. And semantic variance is basically the opposite of that. So, because in principle, we want it to broaden out, exactly.
Susanne
Okay.
Max
But not so much either. So, we don't want a LLM persona from auditing to suddenly be asked about quantum physics. We don't want that either. But that's how we can measure it. How far it goes away, that it goes away. And that was a first serve for me, so to speak.
Susanne
So a bit like in a podcast, when you have a central theme and then you turn left and right. And you want that, to get the breadth out of people, to tease out everything a bit. But it shouldn't go too far either, because then you run out of time a bit. And for me, it's now maybe the view of the central theme that I set myself with my questions. And with AI, it would then be AI itself that has to control that a bit with when it diverges, because it's readable. Right? Misinterpreted? Gone too far? Fantasy.
Max
I also think the comparison is really good. Because the comparison also shows again how difficult the problem is. Whenever I talk about a problem, I mean in the sense of computer science that in principle every task is a problem, that some things, I don't know, can be cast as challenges, but I mean in the sense of, exactly, there is a problem, there is a solution or solution approaches. Now I've lost the thread. But that's why it's so extremely challenging to maintain this thread and follow it. So that's difficult for humans and algorithms, which you use when you write around these models, it's of course much more difficult again.
Susanne
Exactly. And that's exactly what you're working on, to crack that, so to speak.
Max
So just one more thing in terms of outlook. So one of the technical challenges we are currently facing is that it still takes a relatively long time for answers to be generated. And this applies to all models. So not only do you need a lot of hardware resources, but it simply takes time – you know it from ChatGPT and the like – for a question to be answered. But it would be nice if we had a system that could produce almost instant answers, then we could also pre-simulate conversations. And then you could make nice folding diagrams and stuff like that and different progressions and then evaluate which one is better and stuff like that. So that will probably happen sometime soon, that you can make it a lot higher and then you can also test a bit more algorithmically.
Susanne
So we are hoping for the development of AI, where many things are becoming more and more powerful and simpler. Things that were totally difficult a year ago are totally easy now and that also helps you in your project. Exciting, exciting, exciting. But before I torture you any further with these questions, although I think you can probably talk and research about it for hours and days on end, it all sounds super exciting. You said you have a second part, you want to provide the knowledge somehow afterwards. What happens after the conversation with the knowledge? Now you have a system, ideally at some point you will have managed to ask the questions in the right breadth and depth so that you can access the experiential knowledge. And what does it do with the experiential knowledge?
Katharina
Exactly, that's, as you say anyway, Susanne, actually the second part, which follows on from that and which is actually currently very, very open. So you can imagine, I think, if you really assume that you can secure this knowledge well, that it is of course super easy to do whatever you need with it. So whether it's for use in the classic sense for training materials, for example, or for smaller formats. We have already tested this extensively in our first pilots, for example, by saying that you simply make small how-to videos. So, to give a specific example, Max already mentioned it before, we looked at a persona from the field of auditing and tax advice, and we looked at a persona from the field of pharmacy, so to speak, a pharmaceutical representative, because we thought that these are simply two very exciting areas that have to keep up to date all the time and we asked them, so to speak, how they really kept up to date in the course of their professional lives, how they stayed on the ball professionally. Then we simply got five or six key points out of it and then used that again through other AI tools to make small educational videos, so really two- or three-minute videos in which this person also appears and where she simply gives her colleagues the tips she had in store.
Susanne
And there you were already active again as people. That is, you then looked at it, analyzed it and then extracted the key points. Or did the AI already do that?
Katharina
Well, on the one hand, we had the AI do that too, so to speak, and then we created the common thread and the script, just like that, a basis. But then, of course, we went over it again, as I think it is still necessary everywhere, that the human hand is involved.
Susanne
Maybe we don't want to get rid of them at all. I'm always a bit afraid for my profession. But Max, when you look at it from a very, very technical point of view, I think you don't really care about my profession and you look at what technology can possibly get out of it. Do you think that AI can get something out of it and then in the future, on its own, then there is this experiential knowledge now, we have that and then it automatically makes some kind of learning videos with an AI avatar or something like that? Do you think that's possible? Or am I crazy?
Max
Nah, I think it is. That's what's coming. The question is how to control it. Because the big challenge with generative AI in general, especially in its current state, is that, I think, the results are usually good enough. So what comes out of it is somehow good enough. But the question is, what happens if you want a different quality? And then it is already so with image generators, so you can spend hours, that somehow the first result that comes out is quite nice and then you want to have something changed and then it becomes difficult. Then you yourself would have been much faster in the end. And I think that it will be like that for quite a while, but it will probably change a lot. Especially when it comes to whether it is just good enough. And then, well, I think the question is that then... Then maybe it's like, I don't know, I can't think of a good comparison right now, but on the one hand there are demanding, meaningful, pedagogically adept learning contents and on the other hand there are things that simply have to be done, so to speak. I think that's the short-term and in the longer term it's about looking at it. I think that's definitely the direction and it will keep getting better and better. But I can't say when that will happen.
Susanne
Well, just off the top of my head, if you describe it to me like that, and that's really just off the top of my head, what I can definitely imagine is that if you have some kind of facts, experiential knowledge, descriptions or something, it is then possible to provide small information nuggets or something similar. And now we are getting into learning didactics a bit, or Katharina is getting into our topic. Quite often, however, a framework is still needed. So one thing is the information I give and the other is really making learning out of it for people. And that's when it gets exciting, because a lot of learning also happens through self-directed learning via information that you absorb. This means that a gap could really be closed and input created for my colleagues and me, which we could then fall back on, so to speak. So it's a very, very exciting part. Now, I recently read a post about the Knowledge Safe, and someone asked a very interesting question. He said, it's nice that you now want to tap into the experiential knowledge of all the people who work in the enterprise, but we live in a time when the half-life of expertise is not that great. And yes, how do you actually go about it, have you also thought about the fact that the knowledge that has been retrieved might become obsolete at some point?
Katharina
Exactly, I think that's one of the questions that naturally arises immediately whenever you're dealing with preserving knowledge somewhere. And yes, of course, we've discussed it a lot, but we think and we see, above all, the big difference between our work and to continuously adapt this classic factual knowledge, which organizations are of course strongly committed to anyway, to include it in training courses and to keep it up to date anyway, that this is covered by this part in a form and that we, just as experiential knowledge inherently is, we want to access this experiential knowledge, which is often built on many, many years of experience, on purpose, because a large gap actually arises that often simply fizzles out and is lost the moment the employee leaves the enterprise or rotates out of the project. So that is always a central and important question, so to speak, but here I would almost classify it as rather secondary or as rather... Deliberately put aside, because it is about something else at the core.
Susanne
Yes, I think that before you can talk about the fact that experiential knowledge is perhaps outdated, you first have to make it available at all. And then maybe that's the second step that comes along with it. Exactly.
Katharina
Nevertheless, it is perhaps still important to mention that it is of course the case that the knowledge we want to collect here also needs to be quality assured. And in the next step, yes, then absolutely, right now we have cases where, of course, many enterprises are starting to create their own chat GPT, where you then also pour this knowledge in here, but only to the extent that it is still up to date. That means that in some form or another, you still have to do quality assurance anyway.
Susanne
Yes, that is an exciting part, because each of us has his or her own view, but is my view also the one that reflects my enterprise? Yes. Is that it?
Susanne
Max, you wanted to say something, then we went over it.
Max
All right, well, I would basically go into a very similar point, that from a technical perspective, the question is how to combine knowledge from different sources. So from knowledge sources that were created at different points in time, that's one issue. But the other issue is also what you do when they resurface. So that's also, let's say we have an organization where 20 people are interviewed in a department about a certain topic because it concerns all 20 somehow and the point is to find out how they implement something in their everyday lives and all 20 give slightly different answers or completely contrary ones or whatever. So how do you deal with that? And I think that's the exciting question for this second part of how to process the knowledge that you've then externalized. And there is a classic way that would be an uncomplicated solution at first would be a chatbot that then classically, so that would be a document-based chatbot that simply understands all the conversations as a documentary. But on the other hand, something like that might also work. Something like working with semantic search. Because that, I think, I also think that very, very often it is also the case that even if a chatbot is expected, a classic search mask would actually help much, much more. And it's just, just the information in which and the conversations were talking about that that also helps a lot. And then you can see how different people have rated different processes. But in principle, that depends very much on what the expectations of the result are.
Susanne
I just imagined that now that you've described it that way, I was talking to a colleague about it today, this is something really weird, but she tested a bit with the chat GPTs of this world and picked out recipes to see how it could work and just tried out the different options, whether I wanted a really down-to-earth meal or a five-star dinner and then created a recipe from it. And I said, I think it's much nicer to say on Google, these are my ingredients and then I get these recipes from 50,000 provider pages and then it says that this is maybe from one page and this is maybe from another page and then the recipes are in there. And when you describe it like that, I imagine it to be something similar. Tell me if that could be useful. I search for a topic and then maybe the names of experts or something like that will come up and then the expert says, he said this about it, he said that about it. Is that what I have to imagine?
Max
For example, I really like the picture. What I find particularly nice about the picture is that it assumes what you have and what you make of it. That's why I like semantic search so much. I think it's actually technically more exciting than generating answers, but really how to find specific information. And that is also a development that has been going on all the time in recent years. And the best-known things that language models now make possible are more likely to be chatbots. But this semantic search, that is, really searching for meaning, that is, being able to map meaning in lines and compare, that is, being able to mathematically compare the meaning of entire sentences, that is so crazy. I still can't get my head around it, even though I've been dealing with it for years now, because it's just, well, I used to come from the humanities, and just for archive work, when I just have a huge archive and can just search for meaning and don't have to look around to see where information might have come from, who might have mentioned it, I have to somehow work my way through a thousand works. So that's really, I think that will be a much, much bigger change for some areas than just a chatbot.
Susanne
Yes, that's something that I always found really exciting about AI at the beginning, because the Large Language Models, which we mentioned at the beginning, are just modeling language. That is, what they convey in terms of content is irrelevant to these models. So you have to be aware of that sometimes when using Chat GPT. It writes nice things and I put the meaning into it, but what we want is to convey the meaning. And maybe we don't care about the form that much. So maybe not in the end, but roughly speaking, we're still a bit open, but we really go into the meaning. Ah, extremely exciting. I think it's such a cool topic, what you've chosen. And I still wonder, it can't be that we at tts were the only ones who had this idea. Are there others who had a similar idea, who might take the same approach as you or different approaches? So do you know anything about that?
Katharina
Yes, well, I think what can be said is that there are approaches that we are simply flooded with information or expert debriefing guidelines, some of which are already being created with AI support, or where expert debriefings are accompanied by AI to some extent. What we often observe, although I should also say that we certainly don't know all the different products here, even though we try to stay up to date. But what we notice is that many have the approach of conducting interviews, for example, in an automated way, but with pre-defined questions, so to speak. Or then, for example, for each job description, you simply have a pool of 50 to 100 questions or so, where you then know, okay, if I have this and that job level and job description, I go in with the questions. And that's what we consciously don't want to do and where, as we've already described, we just want to generate the questions during the conversation. I would say that is, I think, the biggest difference we've encountered. I don't know, Max, if you have similar observations or others?
Max
Well, as I mentioned, the literature is pretty much at the beginning. I haven't really found anything in that direction that uses language models in particular. And I think that's one of the biggest points in that regard. So there are large language models that have existed longer than ChatGPT, but it takes that broad impact that you can program with it. The hype started with ChatGPT, and since then, the question of what else it could be used for has also arisen. And it's only two years old. So it's only going to be a year old next year, it's just not that long. So that's why, and in research, for example, it just takes time for results to be published. Therefore, I can imagine that work is being done on it right now, because the core problem of something like automated interview planning affects a wide range of areas. But currently, at least as of June 2024, at least the last time I did some deeper research, I can say that it is new. Especially the idea of a truly completely automated system that also interactively responds to what has been said and guides the conversation.
Susanne
What I just had as a thought is that we are assuming that a conversation is taking place and that we are thereby giving up this implicit knowledge. Another approach, a really stupid idea on the side. Actually, I always enter my knowledge into the AI normally, but if I ask questions at Chat GPT or something, are there also approaches to getting the implicit knowledge out? Is it the same or is it just a thought that just occurred to me?
Max
Would definitely be interesting, so that we, so to speak, so maybe two levels, so something like evaluating the questions, so something like the frequently asked questions, that alone, I think that's a slightly different topic, but that you can also identify more specific needs from that. Can, so what it takes for example learning content, apparently, and there you can act again differently, so just when you're working with chatbots, so there are still rule-based chatbots that work with quality-assured knowledge, and just that, so to speak, as, so collect questions that arise so, then check the answers, then see if these questions... questions come up more often or not and to process them differently again. So that's also something we're looking at in our working group. But that's one thing, and the other thing is the topic of this implicit knowledge. So that comes, so if I enter something into ChatGPT, for example, it doesn't have any further influence on the model at first. But that, well, that's sort of, so I throw in a text and get a text out, but the model itself doesn't change at that moment, so to speak. And respectively, well, that's at a certain level of training and it stays at that. But the data, well, Open AI says, I think, that they don't collect that data. I don't think that's a word. So definitely, that's like gold for them. I think that's one of the reasons why it's still free. But then further training sessions arise from that. So you can then use that to train new models. You can then adapt them further and so on. And then again, exactly these questions that come from people are ultimately their data gold.
Susanne
Okay. But that's really a different topic from what we're dealing with in our system and our Knowledge Safe right now.
Katharina
Susanne, maybe I could just add a brief comment on that.
Susanne
Yes, please do.
Katharina
Yes, because that is often what we experience in customer conversations, so this question, yes, I can also just use ChatGPT to prompt, so to speak, and just give it this framework, in terms of, please interview this person now, just try to get the experiential knowledge out, and, and, and. And I think it's perhaps also interesting to realize that we then have the case here that, clearly, in the moment when we enter the prompt, it's all there and then you can, so to speak, get the questions out. But in fact, as Max has already said, it is not really a self-referential system, so to speak, that then also keeps track of things, but it has then also disappeared again. That is, in that sense, it is to be seen from that direction, again unsuitable for conveying that.
Susanne
Exactly, so it's just a system that asks me questions, but they don't take into account all the topics you discussed earlier with the topic, wait a minute, semantic variance and so on. All these topics are then not included at all.
Katharina
Right, if only because it's just not stored sustainably in that sense and you actually have to start all over again and, and, and.
Susanne
Okay, very, very interesting. Are there any points in the future, any trends or any developments where you say that they contribute positively to our research project?
Max
Lots. Lots and lots of very exciting developments at the moment. One thing that comes to mind spontaneously is Visual Language Models. This is a related family of Large Language Models that are built specifically for image data. And these are, for example, super exciting for all work that involves images. So for example, for those who need circuit diagrams or surgical drawings, if that exists. I'm just...
Susanne
X-ray images.
Max
X-ray images, exactly. In such large plans, architecture, drawing, whatever, everything that concerns this. Or the most diverse types of evaluation. So where a completely different kind of knowledge is simply needed, than can be tapped purely verbally. Because that is the modality that we are currently dealing with, is language first of all. And what is missing is the image. Because a lot is not discussed through language, is not transported as well as it is through simple classical pointing. So pointing is often much, much, much cooler and faster than explaining it at length. And another thing is the efficiency of everything. That's also my big topic. So efficiency also in the sense of something like environmental impact. That is also a general topic, but everything that works with power models is, let's say cautiously, optimizable from an environmental point of view. But there are also approaches for specific use cases, models can be made smaller and they are getting smaller. Last year, there were still two opposing trends. On the one hand, models were scaled up more and more, and on the other hand, models were also getting smaller and smaller, because in the end, nobody really wants that. So if you want to work with it, you really need smaller models that you can ideally just run on a conventional laptop, without somehow setting up a graphics card data center for it.
Susanne
I once heard that they consume as much electricity as entire cities and such. Is that something you're counting on?
Max
Yes, absolutely. Well, it's really, I think it's pretty disastrous that it's been announced that Microsoft is now building power plants just for data centers, that kind of thing. So that's not a nice development. That's not cool. So it should be done differently. That's just... So not entirely relevant to the new Knowledge Safe topic, specifically now, because the usage figures there don't go into the millions either, where it just...
Susanne
Well, we don't compare ourselves to Microsoft, do we?
Max
Exactly, but these are topics that you also have to consider there, so how such a system still remains lean and efficient so that the footprint doesn't go completely off the rails.
Susanne
Ah, okay. Yes, very, very exciting. Katharina, do you have anything to add? That sounded very comprehensive.
Katharina
Yes, definitely. I only remember now, but I think Max can give us more information about that. But Max, about the one lecture you were at just recently, or about the recognition of European dialects, the European dialects. Or Max, have you already said that? I'm not sure now if I missed it before. I think that would be another interesting aspect, because it is particularly important for us right now in terms of speech recognition and such, because we are active in the attic area, after all. And maybe you can give us a little preview of that again.
Susanne
That's exciting because you have such a beautiful Austrian dialect.
Max
But in any case, this is also a bigger topic in general in the development of language models that deal with text and also simply with the spoken word: the question of what they can actually process, that is, which language they can process. The models have already become a lot more flexible. That means, I really mean the pipeline from text-to-speech again, which, funnily enough, is also abbreviated TTS. But there, that's why I was a bit confused a few times. But yes, these TTS pipelines are still by far the best in English because that's what they're trained for. But in German, for example, it has become much, much better in the meantime. So there are already many usable models, but then the question arises as to which German. And that is within, so there are dialects, there are accents, there are speech impairments. There are many, many, many variants of speech. So I say that as someone with an accent and a Saxon background. So far, most TTS systems have despaired of me. Yes, that's why I can always test how it is at the moment. But exactly, there is a lot of research going on in this area as well, because the demand is simply great. So that also for languages that are simply smaller. For example Bosnian, Serbo-Croatian, which is a smaller language for the... but now very targeted models have been trained. For example, I think that's pretty cool too. So that is also coming more and more and especially European languages are covered more and more. And there is also a model coming out soon from another Fraunhofer Institute that currently covers, I believe, 25 European languages and should be able to handle dialects much better next year.
Susanne
That's exciting. So you've told me about three things now. One was this AI in the image context, then the topic of energy, and now also this linguistic diversity that we all have. So I think there's a lot more to come, and maybe it will really have an impact on our Knowledge Safe. Great. I'm actually blown away by all the things you're telling me. It's super exciting, which I haven't yet. And whenever I say “super exciting”, it means that I'm still working on it. And on Monday I'll call Katharina and actually say, “Hey, I came up with this, this, and this.” But what I've been asking myself the whole time is actually... On the one hand, I sometimes have the feeling when I listen to you that it's something you're already testing on people, you can already try it out, you can already buy it. And on the other hand, no, no, there's still so much left open and there are still so many topics that we want to clarify. What is the current situation there? What steps are you currently planning? What might be the short term? Is there anything you want to share with our listeners? Is there anything?
Max
Well, there are quite a few things we are working on. Nevertheless, there is already a kind of product that is not yet fully automated. But Katharina can probably say a lot more about that than I can, because I usually have the research view. That means I'm also interested in the things that don't work so well yet. So that's exactly where I think, cool, that's exactly my thing, we can talk about that, and from that point of view, I can definitely say that it's already quite concrete and that even the steps that are not yet automated. For example, we have had a feasibility study that has also provided the first results, which are already good to work with. What is not yet covered here is the second part. So the first part is to externalize this knowledge and first show that it is possible to build an automated interview system that pays attention to certain things, such as semantic variance. That is the state we are at now. And the next point is... is the question of what you do with it. So, where we were talking about it earlier, for example, individual knowledge nuggets, however we formulate them, or introduce them into chatbots. Well, there are 10,000 options. And that is basically also the nice point where the project currently stands. Because the point is to work with very specific use cases. And that means that it can be looked at again in a very customized way. And if, for example, the requirement is that something like a knowledge graph should come out or that work should be done on the image material, or whatever it is, that this simply opens up a great many possibilities again, especially to examine very nice things again and then get them into products.
Susanne
I think that's very nice. It also fits in with what you said earlier, that for me a problem is something beautiful because then I can find a solution to it. And that's reflected in the way you talk about the topic. I'll let you, Katharina, translate that into the business world. What does that mean for us now?
Katharina
Exactly, I think Max has summarized it very nicely. So, in essence, that the product as it is, we have already taken many, many steps, so to speak, and have already taken many steps forward. Also, with nice, very specific use cases, which was of course important to us from the very beginning, to simply get partner companies involved and test it and also test it on us and so on. And that is precisely the nice point, to see how much is happening everywhere and, as already mentioned, many customers now have their own chat GPT in-house, where the demands are suddenly also going there, so to say, in addition to the other things mentioned, and we then also want to integrate and connect that there. That means that the challenges for the objective are very, very diverse and change almost daily, but that's a good thing because we are currently still a very agile project in some form or another and so it is still important for us to work with many, many different industries and to test as many use cases as possible, on a smaller with maybe two to five interview subjects, on a larger scale, where you might say, you might take 10 or 20 people with you, maybe look at one position or even take two or three departments and look at it between departments, in order to be able to ask targeted questions again. That means, the playing field, so to speak, is currently still very large and we are trying very, very hard to respond to the requirements with which enterprises approach us. And so we are also open to perhaps everyone who is interested in joining in, participating, helping to shape the project, or perhaps getting in touch with us again from a scientific point of view or simply out of interest. We are also very, very open to this, of course. So, we have already had a lot of that with other partners who have also said, hey, they have an internal AI task force and just want to know what we are doing here. And so this is just a very nice, open and very, very flexible project in some form, just as the inventor intended.
Susanne
Oh, that sounds so great. I'll volunteer as a tester if you need someone.
Susanne
What you've described today sounds really, really super interesting. And I somehow have the vague feeling that we've only scratched the surface a bit and you're so much smarter than me. I really don't like that feeling at all. I would much rather have gained access to your experiential knowledge, but there wasn't enough time for that. I hope to hear more from you as developments progress. Maybe I'll check in with you every six months or a year to see if there's any new development and then we'll just talk again. It was a wonderfully pleasant conversation we had with you. I would like to say a big, big thank you to you, Katharina, anyway, but we see each other regularly, but especially to you, Max, for coming here and explaining all this to me, from whom I am always the DAU, who exists in all these topics, but always curious. I'll let you have the last word. What else do you want to say to the people outside? I'd say goodbye, or rather, see you later. What is your final word for our listeners?
Katharina
Yes, off the cuff. Good question. Maybe what I said before. So really, anyone who likes, who is interested, to whom we were able to make the topic of AI a little more palatable or simply arouse curiosity, is welcome to contact us.
Max
I would like to suggest that there are more funding programs for efficient AI systems. That would be very cool. We can apply for that right away and then see how to make the whole thing even more efficient.
Susanne
Great topic. Thank you very much and see you next time.
Katharina
See you soon. Bye.
Outro
By the way, have you subscribed to us yet? You can do that wherever you prefer to listen to your podcasts. We look forward to your feedback and, above all, to interacting with you. How can you reach us? You can write to us on Podigee or follow the LERNLUST podcast on Mastodon. We also exist as real people on Mastodon and LinkedIn. So tell us what you like about our podcasts and where we can improve. Until then, I look forward to seeing you in the next episode of the LERNLUST podcast.
Show me similar content
LERNLUST Podcast Knowledge Transfer Knowledge Management Artificial intelligence